August 2019
tl;dr: End-to-end pseudo-lidar training with 2D/3D bbox consistency loss.
Overall impression
This paper’s main idea largely overlaps with that of pseudo ldiar. The main problem with pseudo-lidar is the noise (i.e., depth inaccuracies, long tails) in the reprojected 3d point cloud due to blurry boundaries. Pseudo lidar ++ proposes to use sparse depth measurement to alleviate this problem, while this study uses 2D and 3D bounding box consistency (similar to deep3DBox).
However there is a major problem with the current approach. The idea of trying to predict a correct 3d bbox from a noisy point cloud is not optimal and the 3d box prediction get even “contaminated” from the 2d-3d bbox consistency. A better way is to finetune the point cloud generation process as well. This requires propagating the depth gradient to the depth net. –> see depth coeff for a solution!
Pseudo-lidar++ tackles this fundamental problem and achieves better performance, but it requires supervision from sparse depth measurements.
Note that DORN’s training data overlaps with object detection’s validation data, and suffers from overfitting. Both pseudo lidar and pseudo lidar e2e suffer from this problem. According to the ForeSeE paper, if the validation data is excluded from the training of depth map, then PL’s performance drops from 18.5 to 5.4 AP_3D at IoU=0.7.
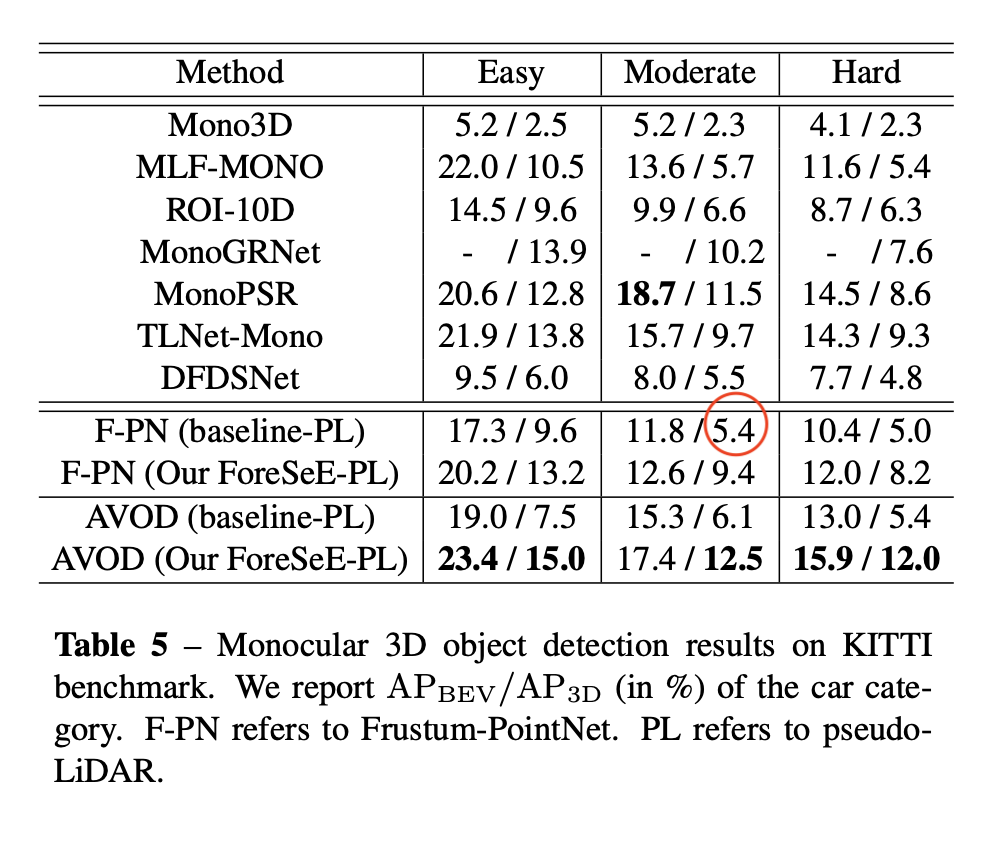
Key ideas
- Limitations with depth sensors
- Stereo cameras: calibration and synchronization
- Depth camera: limited working range (<10m)
- Lidar: high cost
- The noise in pseudo-lidar point cloud
- local misalignment due to inaccuracies in depth
- long tail “bleeding edge artifacts” from blurry object edges in depth maps
- Architecture
- Depth generation: pretrained model
- pseudo-lidar Generation: use extrinsic matrix [R t] to transform to body frame (as 3d bbox annotation is in body frame)
- Frustum pointnet for 3d bbox estimation
- instance mask instead of bbox to extract frustum (this is to curb long tail)
- point cloud instance segmentation
- Adjust 3D bbox to align with 2d bbox
- 2D-3D bbox consistency
- Convert 3d bbox to 8 corner representation and get MBR (minimum bounding rectangle)
- During training, 2d-3d bbox consistency is used to regularize the 3D bbox prediction. During inference, the consistency is solved as an optimization problem, similar to deep3dbox.
Technical details
- Many components seems to be used as pretrained off-the-shelf modules, such as the depth estimation net and the instance segmentation net.
Notes
- Q: Can we incorporate the limited length of cars as priors to regularize the projection? Maybe refine the depth map using the 3D prior info. Or can we skip the edges?
- The main problem of the paper is that the 3d bbox prediction is on top of a noisy 3d point cloud. The detector is definitely not optimal. Maybe detach the gradient from the 2d-3d bbox consistency is a better idea.